What makes for higher quality piano stems?
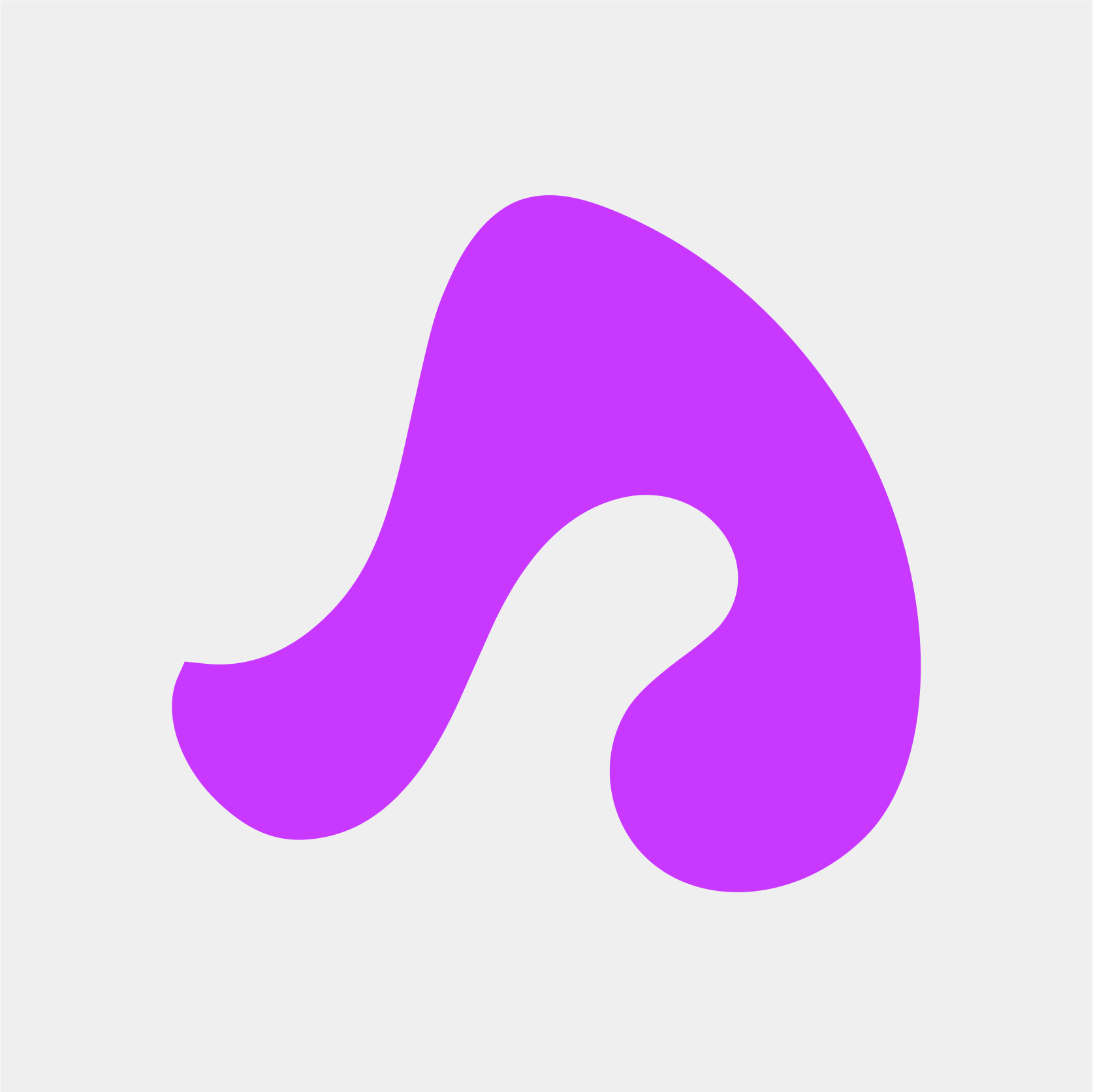
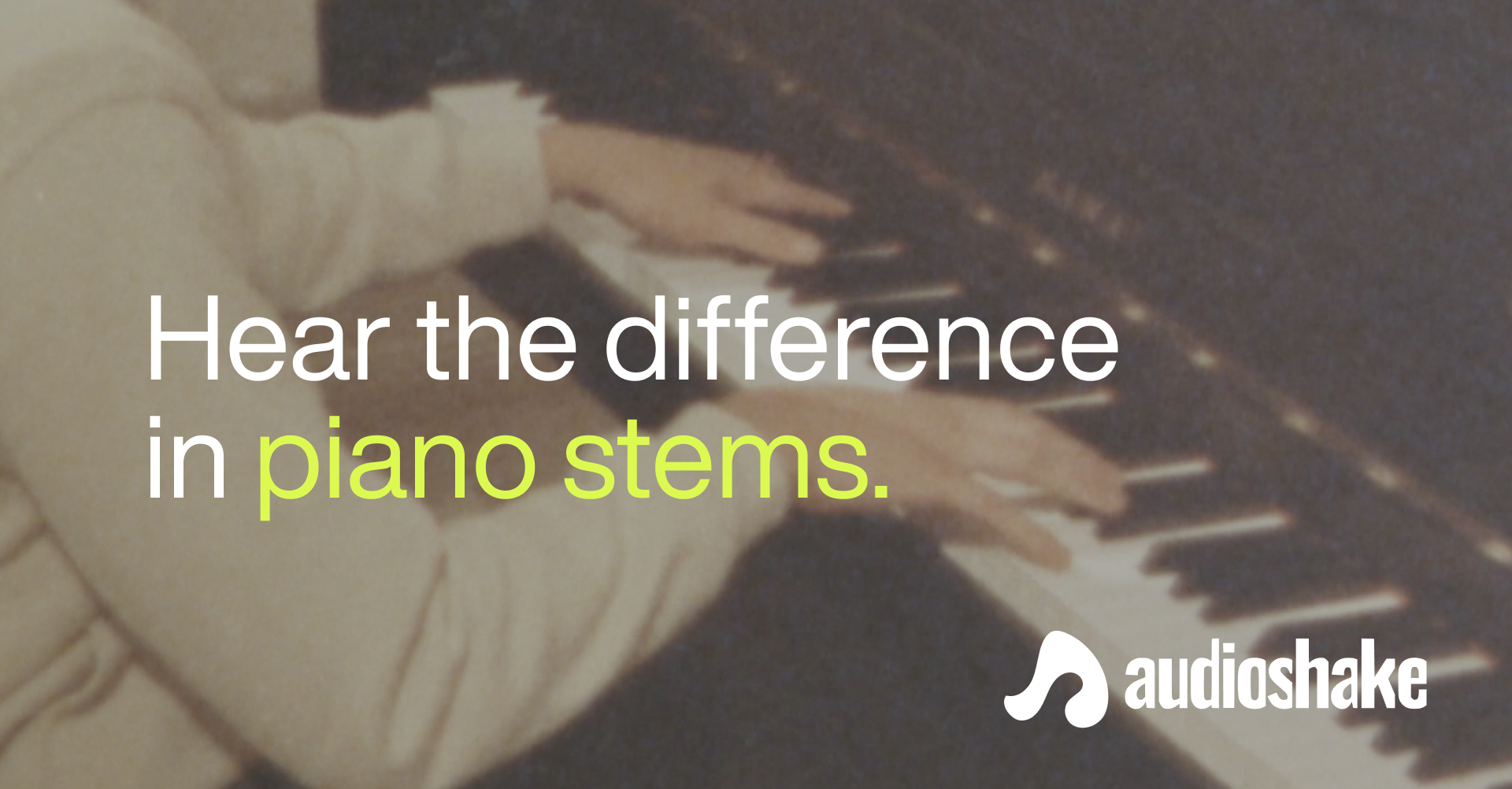
To some ears, the difference in quality between our earlier and new models may be imperceptible. Then, there are times when the updates to our models are so great that we can both see and hear a huge difference.
Our team was proud to launch our new piano stems last month after seeing our models separate even cleaner stems with well-balanced tones across a wide range of pianos. From classical grand pianos to digital pianos, our piano isolations were able to capture clear highs across genres and performed well on expressive dynamics.
Optimizing AudioShake models for perceptual clarity
Not only did our latest piano model see a huge leap forward in separation performance, but we also made sure that the perceptual quality has improved significantly as well (here's a bit more about how we think about qualitative and quantitative measurement). Our team crafted a cutting-edge, deep-learning audio model specifically tuned to extract piano and through a fusion of new architectures and pristine clean piano performances could optimize perceptual clarity.
From the classic grand piano–with its majestic presence and rich, resonant tones–to the upright piano, which offers a more compact yet equally compelling sound, our piano model can tackle a wide spectrum of piano sounds.
Another big win for our team was seeing that these clean piano isolations could hold up when separating electronic or pop music. The improvements we made between AudioShake’s 2023 and 2024 stems open up cleaner separation for these genres.
Separating orchestral compositions for AudioLabs Research
We also shared our new models with the team over at AudioLabs (a joint institution of Fraunhofer IIS and Friedrich-Alexander-Universität Erlangen-Nürnberg), who investigated a specific source separation problem by considering recordings of piano concertos - an important genre of classical Western music that has not yet been addressed in the source separation context. The source separation problem involves separating the piano part and the orchestra as if they were recorded in isolation.
“It's remarkable to witness the substantial advancements that deep learning has brought to audio source separation, even in challenging scenarios. During our piano concerto experiments, I was deeply impressed by the performance of the AudioShake system, especially considering that it had never been trained on this type of data before.” – Meinard Müller, professor for Semantic Audio Processing at the International Audio Laboratories Erlangen, Germany
Unlike popular music separation, this scenario presents additional hurdles. First, there is a potential for a significant overlap between the piano and the orchestra in both time and frequency due to shared rhythmic patterns and harmonically related notes. In many cases, the piano and the orchestra even play in unison.
Second, for classical music in general, there are hardly any multitrack recordings available for training, mainly due to the different production processes in classical music, requiring a close interaction between the various musicians when performing. Third, the reconstruction of the sound sources for many different instruments in the orchestra is challenging.
For evaluation purposes, AudioLabs developed a high-quality dataset containing separate piano tracks overlaid with existing orchestral tracks, sourced from the music producer MMO (music minus one). This dataset enables a realistic assessment of source separation systems.
Harnessing AudioShake for the isolations, AudioLabs found that our models yielded the highest quality stems, encompassing both the piano and residual instruments representing the orchestra.